
With the cluster project nextAID³ – Next-Generation Al-Empowered Drug Discovery and Development, Saarland University is applying within the framework of the Excellence Strategy of the German federal and state governments. The cluster, which has grown out of the NanoBioMed research focus, aims to break new and innovative ground in AI-driven drug discovery and development.
Various diseases and resistance to antimicrobial agents pose a threat to the health of a growing and ageing society. Drug development is expensive and takes too long to make the new drugs needed available in the short term. In our Cluster of Excellence nextAID³, we aim to lay the foundations for combining active agents more efficiently by combining local advances in Artificial Intelligence (AI) and experimental research in novel ways.
This synergistic approach unlocks the potential of three important research areas that offer less incentive to the pharmaceutical industry: new natural products with broad biological activity, new anti-infectives to overcome microbial resistance, and unconventional targets as the basis for novel mechanisms of action. We are harnessing the potential of AI for hit identification and multi-parameter optimisation to advance drug discovery of synthetic molecules and natural products. Optimised drug delivery is supported by innovative approaches.
In our young nextAID³ consortium, we conduct interdisciplinary drug discovery. We build on the visible strength of the UdS with its university hospital and non-university research institutions. The interdisciplinary research team is led by Professors Anna Hirsch, Martina Sester and Andrea Volkamer. On the university side, pharmaceutical research, IT research and biomedical research at the Faculty of Medicine and Saarland University Hospital are involved. Scientists from the Helmholtz Institute for Pharmaceutical Research Saarland (HIPS), the Helmholtz Centre for Information Security (CISPA), the German Research Centre for Artificial Intelligence (DFKI) and the Leibniz Institute for New Materials (INM) are also involved.
We are supported by the existing research infrastructure and the recently founded PharmaScienceHub, a sustainable cooperation platform in a new research building. Key structural goals of nextAID³ are to provide interdisciplinary competencies for young researchers, to ensure equality and diversity, and to create transparent, family-friendly career paths. The transfer of new technologies and molecules to industry benefits from a strong culture of successful start-ups at the UdS.
Speaker
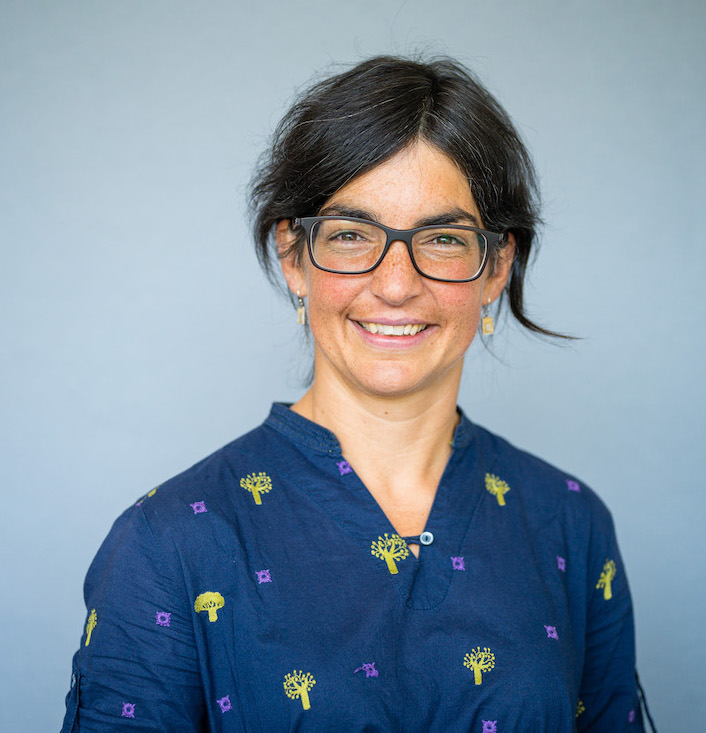
Designated Speaker
Anna Hirsch is Professor of Medicinal Chemistry at the UdS and heads the Department of Drug Design and Optimisation at the Helmholtz Institute for Pharmaceutical Research Saarland (HIPS).
Die Hirsch-Gruppe verfolgt eine strukturbasierte rationale Designstrategie, bei der sie sich auf biologisch relevante, häufig wenig erforschte Enzyme, Transporter und Regulatoren aus Bakterien oder Parasiten konzentriert. Die Gruppe verwendet diverse biophysikalische Methoden, um Wechselwirkungen zwischen Substanzen und ihren Zielproteinen zu untersuchen, und setzt zahlreiche in vitro- und zellbasierte Assays zur Evaluierung neuartiger Anti-Infektiva ein. Basierend auf diesen Ergebnissen erfolgt die Multiparameter-Optimierung der Wirkstoffe.

Martina Sester is Professor of Transplantation and Infection Immunology and Head of Department of the Institute of Infection Medicine at Saarland University.
Her Department of Transplantation and Infection Immunology was founded in 2009 as an interdisciplinary department to combine basic research with patient-oriented research. The scientific focus is on the regulation of cellular immune responses against clinically relevant pathogens and against donor tissue after organ transplantation. Another focus is on monitoring infectious complications under immunosuppression and on quantifying the effectiveness of immunosuppressants.
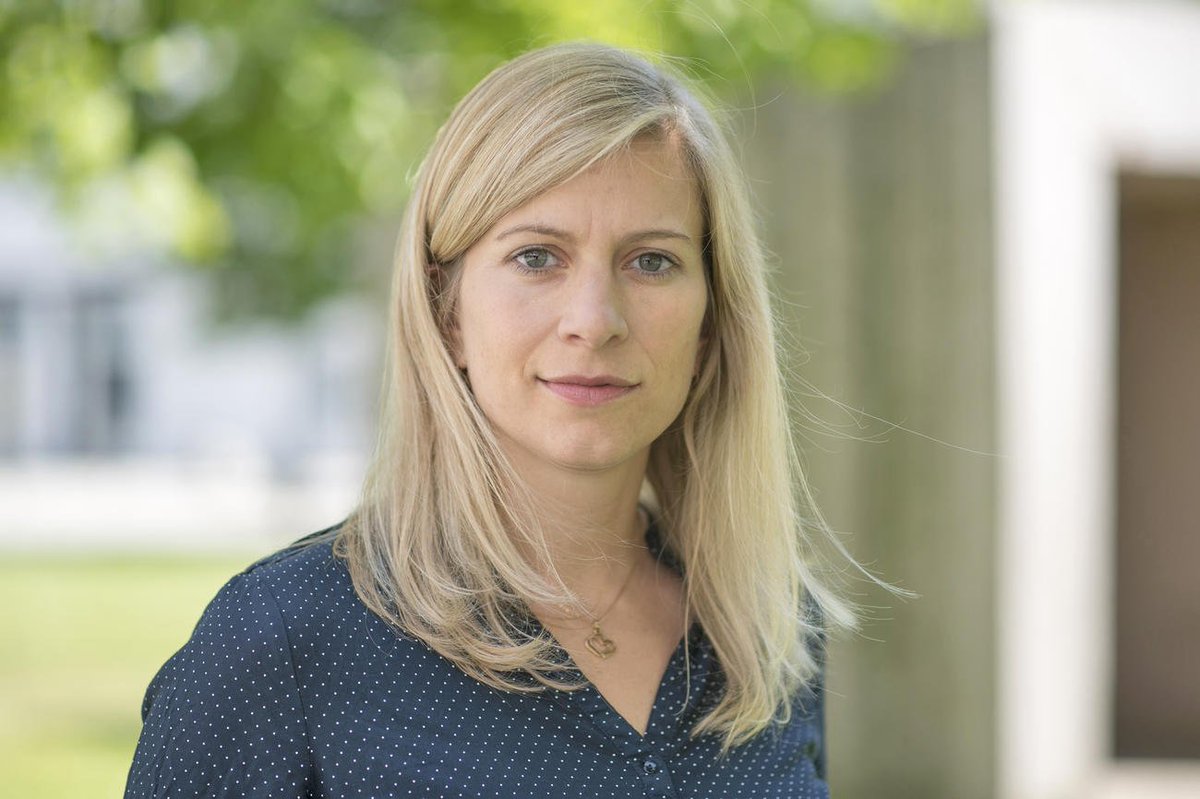
Andrea Volkamer is Professor of “Data Driven Drug Design” at Saarland University and an associate scientist at the Helmholtz Institute for Pharmaceutical Research Saarland (HIPS).
Prof. Volkamer's research focus is data-driven drug design with a focus on method development and application. The group develops methods at the interface of structural bioinformatics and cheminformatics with a particular interest in structure-based machine learning approaches applied in the context of computational drug design, especially kinase research, and in silico toxicology.
Participating institutions
Saarland University (UdS) is one of the medium-sized universities in Germany and a member of the German University Alliance (UA) 11+. As a comprehensive university, it covers a broad spectrum of subjects in teaching and research. The focus areas of computer science, NanoBioMed and Europe form the core of the excellent research.
The research focus “NanoBioMed – Life and Matter” brings together natural sciences and medicine. The focus is on interdisciplinary and innovative research in the fields of medicine, pharmacy, life sciences and bioinformatics.
The departments of life sciences, chemistry, computer science, materials science and engineering, pharmacy, physics, systems engineering and clinical and theoretical medicine work closely with the inter-faculty centres for human and molecular biology, bioinformatics and biophysics, as well as with various non-university research institutions.
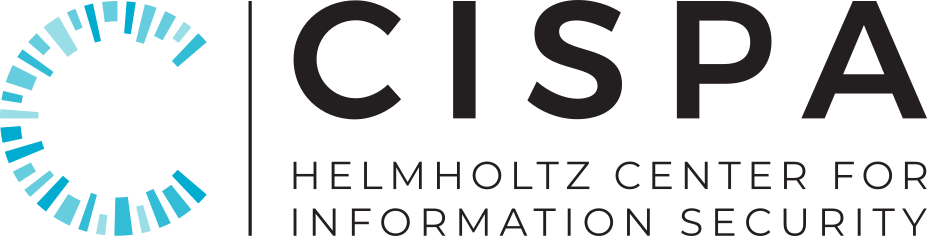
The CISPA Helmholtz Center for Information Security is a German national Big Science Institution within the Helmholtz Association. CISPA is dedicated to cutting-edge basic research combined with innovative application-oriented research in cybersecurity, privacy and artificial intelligence.

The German Research Center for Artificial Intelligence (DFKI) conducts research on “AI for humans”, focusing on social relevance and scientific excellence in the crucial future-oriented research and application areas of AI.
DFKI is convinced that AI technologies help to successfully address challenges facing society as a whole, such as man-made climate change, social injustice and the fight against dangerous diseases, and is committed to these tasks with great energy. As the largest independent research centre for AI worldwide, it initiates, implements and supports numerous activities to place reliable and trustworthy AI from Germany and Europe at the forefront of international competition.

The Helmholtz Institute for Pharmaceutical Research Saarland (HIPS) was founded in 2009 as one of the first Helmholtz Institutes nationwide by the Helmholtz Centre for Infection Research (HZI) together with Saarland University.
Located on the Saarbrücken campus, HIPS is the first research institute in Germany explicitly dedicated to pharmaceutical research. The focus is on the development of new anti-infectives and their adaptation for use in humans (translational research).
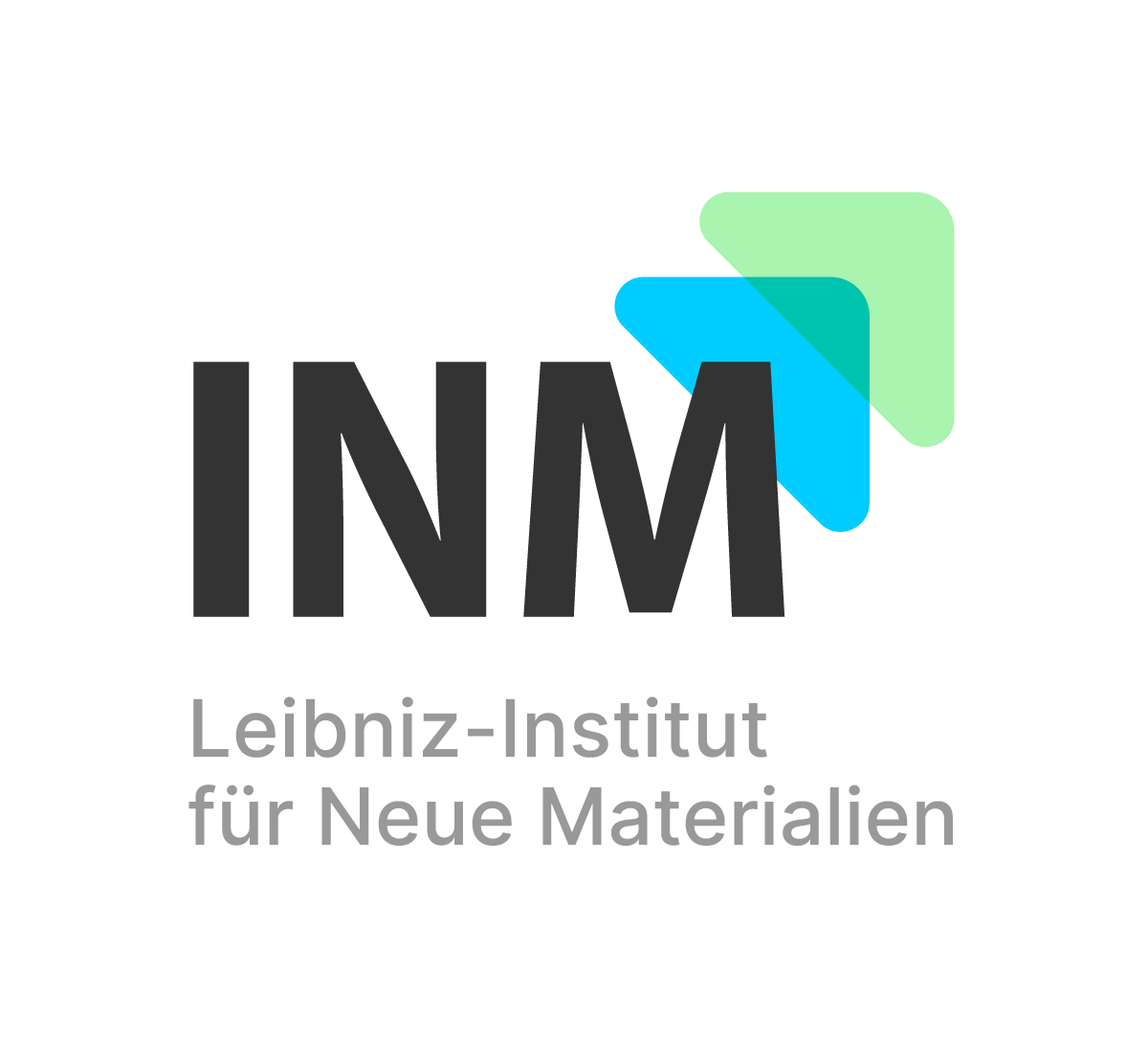
The Leibniz Institute for New Materials (INM) combines multidisciplinary science and materials-oriented technology transfer under one roof. Chemistry, physics, biology, materials science and engineering work together in close cooperation at a high level. A major focus of the work is the transfer of biological principles to the design of new materials, structures, and surfaces.
The results are used in flexible displays and intelligent gripper arms, powerful batteries and efficient solar cells, as well as technologies for personalised therapies and regenerative medicine.